Machine learning algorithms operate in a so-called black box — so the inputs and outputs are known, but how or why an algorithm makes the recommendation it does is not clear. Despite the AI media frenzy, companies and governments are concerned about the machine learning black box. The EU’s General Data Protection Regulation, which takes effect next year, includes a right to explanation clause, or an explanation of how a model made a decision.
The Explainable AI (XAI) program aims to create a suite of machine learning techniques that:
- Produce more explainable models, while maintaining a high level of learning performance (prediction accuracy); and
- Enable human users to understand, appropriately trust, and effectively manage the emerging generation of artificially intelligent partners.
New machine-learning systems will have the ability to explain their rationale, characterize their strengths and weaknesses, and convey an understanding of how they will behave in the future. The strategy for achieving that goal is to develop new or modified machine-learning techniques that will produce more explainable models.
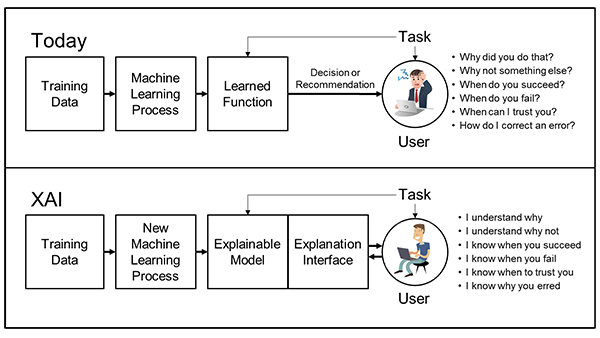
In the context of machine learning over business data, business users are increasingly looking out for features that present the logic or features that lay the foundation for decisions made by machines. For instance, machine learning modules should be able to
- Present observables on why it has decided to propose a certain engagement to a customer
- Understand why a certain product message needs to have different tactical message than the others
- Explain parameters on why an engagement can result in success or result in abandonment
- Explain the basis of a certain prediction or prescription and so on.
In order to maintain transparency which translates to trust among users, Plumb5 machine learning program aims to create a lucid processing model that puts forth the steps of learning, decision making, and automation.
The slide deck below illustrates how Plumb5 Learning model explains or present observables at every layer as to build trust, transparency, and clarity to decisions or actions carried out by the machine.