Delivering personalized messages to users is a daunting task as it involves two intricate processes:
- Extraction of behavior patterns out of vast amounts of unstructured customer interaction data, across all channels.
- Generating patterns across multiple events to predict possible next action of your customer.
To complicate matters, delivering personalized messages when the user is active at any of the touch-points requires you to drive these predictions in real-time. Only then can you deliver messages that have relevance and create the right experience.
To achieve this goal, it is important to set up a system that is capable of detecting patterns and predicting options in real-time, in order to implement a highly effective personalization mechanism for your business.
How does it work?
For a machine to personalize and drive the user to the desired goal path, it would need to match and deduce the next best event and target message that will drive him to the goal path. This computation needs to be done in real-time in order to personalize instantly when the user is active.
This real-time computation would require (a) appending current session behavior pattern to past behavioral pattern of the current user and (b) the deviation of the current user path to the most optimal goal paths in relation to a specific product.
With these parameters, the algorithm calculates the differential to arrive at the next closest pattern leading to the goal. As the user interacts, the algorithm appends the new action and re-adjusts to output the closest probable.
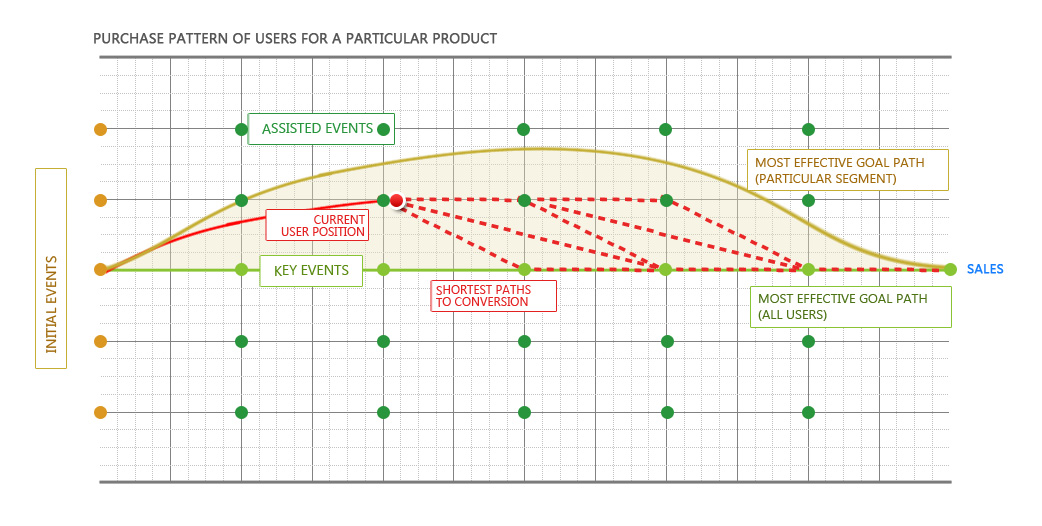
In the example diagram above, the pattern of user interactions is mapped from the initial event to the desired goal path. After analyzing user’s interaction flow for a single product, we arrive at the most effective conversion pattern. To fine-tune our chances, we also generate another effective conversion pattern by just using user interaction patterns, belonging to the segment of the browsing user.
The area analyzed shows the effective probable purchase zone for the given product, which is used to map the relation with the current browsing pattern of the user. Now whenever the user’s pattern is constructed in real-time, the algorithm for matching patterns can quickly locate area coordinates and return the nearest event node to deduce possible pattern extensions.
If there are more than one probable, the automation queues up the probable next events for follow-up targeting, based on the results of the targeted message. The marketer can configure multivariate tests to ensure that the right options are learned and exercised in real-time increasing effectiveness of personalization campaigns.
This automation can drive every user to the desired goal path and can be configured to perform across various stages of the customer lifecycle. This will help businesses to bring back drifting prospects or reduce customer churn with negligible attrition.
To enable effective personalization for your business across all customer touch-points, do get in touch with the Plumb5 Analytics Team.